Three ways researchers are using machine learning to enhance our understanding of the human body
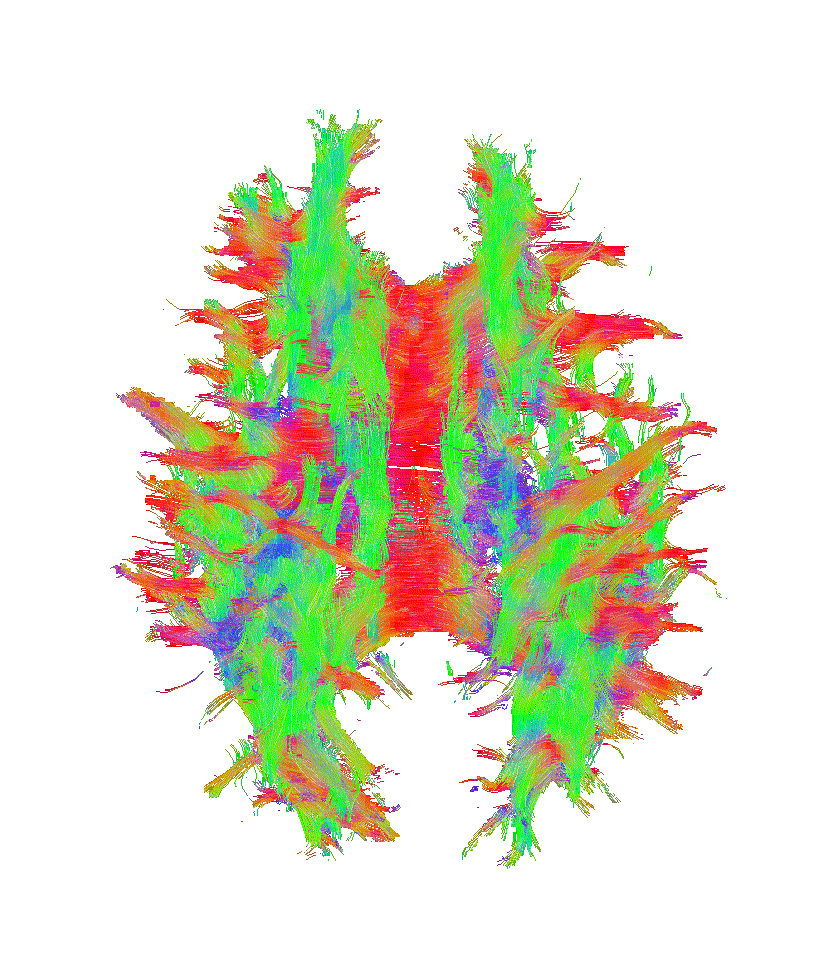
Using technology powered by artificial intelligence, scientists are able to visualize biological processes and gain valuable insights into potential treatments for a range of diseases and disorders
Researchers at Johns Hopkins University are leveraging artificial intelligence to generate clearer images of some of the tiniest places within our bodies—cells, genes, brain synapses. With a clearer view, scientists hope to better understand—and treat—diseases related to aging, genetic maladies, and neurological disorders. Here’s how.
- Visualizing trillions of individual brain synapses to study learning, aging, and disease
Johns Hopkins scientists have developed a method to visualize and track changes in living brain synapses. The study brought together scientists with expertise ranging from molecular biology to artificial intelligence to explore the brain. Here’s what they found:
- In the brain, nerve cells transfer information through synapses. Researchers believe that synapses get stronger or weaker based on life experiences. For example, learning something new might strengthen synapses, while being exposed to illness might weaken synapses.
- There are trillions of synapses in every brain; they are very small, but very dense. Even the most state-of-the-art microscopes can’t visualize them clearly. To better understand how the brain works, scientists literally need a clearer picture.
- Scientists are training machines to generate higher-quality images of live brains based on an “enhancement algorithm” generated from more detailed scans they were able to gather from the brains of deceased mice. With the ability to detect and track individual synapses, scientists can now begin to study how the brain changes over time—as it learns, as it ages, as it is exposed to stimulating environments, and as it experiences diseases and injuries.
The researchers are now using this machine learning approach to study synaptic changes in animal models of Alzheimer’s disease, and they believe the method could shed new light on synaptic changes that occur in other disease and injury contexts.
2. Activating genes on-demand to treat cancer and other genetic diseases
Every cell in our bodies has our DNA, which includes a special snippet called an “enhancer.” That piece acts like an on-off switch to determine what those cells become—heart cells, brain cells, muscle cells. Sometimes the enhancers make mistakes, contributing to the development of genetic diseases such cancer and diabetes.
A team of Johns Hopkins biomedical engineers has developed a machine-learning model that can predict which enhancers play a role in normal development and which play a role in disease development. It’s an innovation that could someday power the development of enhancer-targeted therapies to treat or prevent genetic maladies.
The creation of the machine-learning model included several complex steps— starting with a model of the cell itself, identifying the genes that control the cell’s fate and the enhancers that can influence those genetic decisions. Scientists then used the CRISPR gene-editing system to disrupt or stimulate the enhancers and observe the effects.
Using that data, scientists have made two important discoveries: the precise location of the enhancers that have the strongest impact on genes and the narrow window of time when those enhancers are susceptible to influence.
With that understanding, scientists can now design new experiments to further study enhancers at those precise times and locations. In doing so, they hope to determine which enhancers are connected to certain genetic diseases—and how we might alter them to prevent or cure genetic maladies.
3. Generating “super scans” to understand neurological diseases
There’s a specialized MRI technique that measures how the brain responds to the scanner’s magnetic field. These “super scans” essentially create a 3D map of the brain, enabling researchers and physicians to better understand, diagnose, and monitor neurological diseases, such as multiple sclerosis and Alzheimer’s disease.
Despite its potential utility, very few 3D maps are generated today because it’s so difficult to get a good reconstruction—requiring six different scans at precise head orientations. Johns Hopkins researchers have developed a new algorithm that enables AI to assist in the reconstructions, making the process faster and more pleasant for patients.
The team recognizes the challenges of developing an algorithm to construct an image of something as complex as the human brain. Their algorithm uses an approach called “regularization” to narrow down the number of possible solutions, using prior scan data to teach the model what good brain reconstructions should look like.
The hope is that their machine learning algorithm will make the specialized scans more accessible for clinicians and radiologists by cutting down the time it takes to perform a scan and boosting the quality of the image. The team has made its data, models, and code available as an open-source project for other researchers.