The future of AI in the U.S. and the challenges in sustaining its growth
NVIDIA, OpenAI, and former government leaders discuss the next decade of artificial intelligence innovation and what roadblocks the government should address to keep the U.S. competitive in the sector.
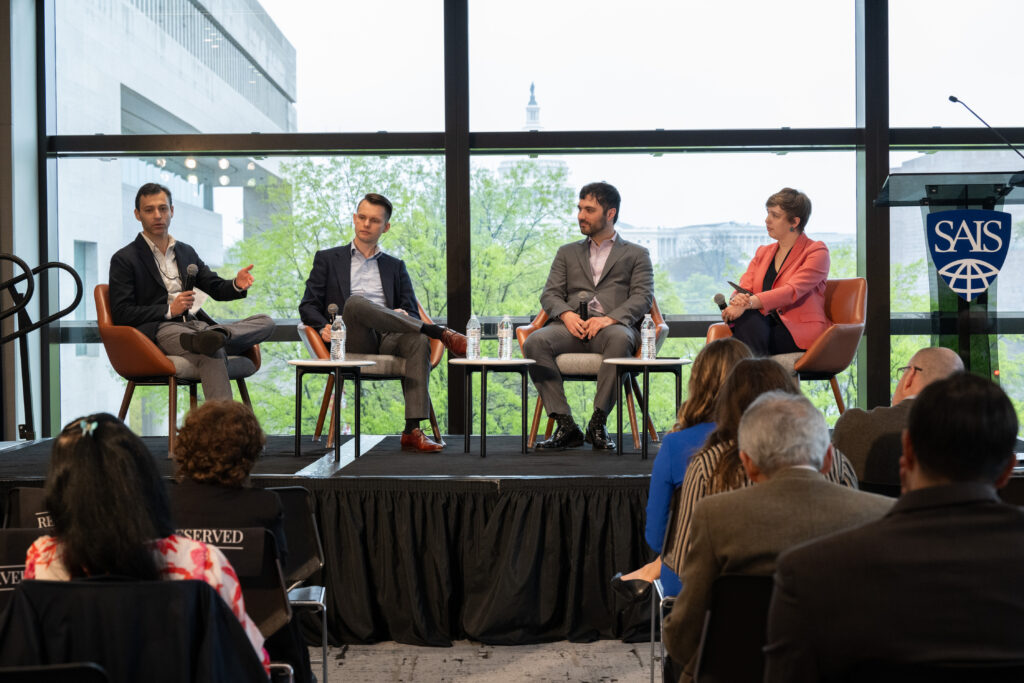
The exponential growth in computing power over the past decade has not only stimulated the global race for leadership in AI innovation but has also heightened the energy and infrastructure demands needed to sustain the technological advances.
The sector is at a decisive moment, and at the second annual SAIS Emerging Technologies Symposium at the Hopkins Bloomberg Center, industry and policy experts stressed that regulatory and economic conditions—as well as labor and supply chain issues—can make or break the United States’ edge in AI leadership as we look to the future.
What does the next five to 10 years of AI growth look like?
If current trends continue, AI will require more physical infrastructure, like data centers, and more efficient energy infrastructure than currently available at home and abroad. This growth trajectory is driven, in part, by the demand from companies adopting the technology.
“We’re at the very beginning of an inflection point, and it is hard to take trends now and just linearly drag them forward into the future,” said Eric Breckenfeld, NVIDIA’s director of technology policy.
Still, there are a few potential shifts and developments that AI experts predict for the sector over the next several years.
Rise of inference. Experts expect to see more demand for computational power for inference, which is the process of running new data through a trained AI model to draw conclusions and make predictions. Most users interact with this aspect of AI most directly when they enter a query into ChatGPT, Perplexity, Gemini, or any other AI model. Inference has also supported the widespread use of China’s DeepSeek AI model, which shocked the world in January when it launched and topped app download charts, as well as other models used by Meta and OpenAI. Because of the different ways inference can be leveraged—whether on a small personal device or in a large data center—it will have unique needs.
Increased energy costs. AI will, without a doubt, lead to a surge in energy use in the U.S., with data centers on track to account for almost half of the growth in electricity demand between now and 2030. This trend has sparked sustainability concerns, but experts noted that greater energy demand could support efforts to secure government funding and attract private investment in better energy infrastructure.
“We don’t have to look at this march toward a base-load demand expansion as a bad thing,” Breckenfeld said of the tension between environmentally conscious energy consumption and AI’s growing energy needs. “It can mean an opportunity to drive better energy technology development. When the demand is growing, that’s when there’s the best opportunities to explore new technologies, because new technologies are expensive.”
Efficient, specialized AI models. Breckenfeld expects to see a shift away from data-heavy foundational models and toward more diverse, medium-sized models tailored for specific uses. Not all models are or need to be frontier models. As the AI market continues to mature, there will be more companies, like DeepSeek, developing models for specific—or narrower—commercial applications across a variety of sectors, such as healthcare and financial institutions.
What are the main challenges to AI growth in the U.S.?
AI infrastructure projects are robust and complex. To operate at a large scale, AI requires:
- Data centers where large numbers of chips are located
- Energy generation infrastructure to power these centers
- Transmission facilities
Each one of these projects faces its own regulatory and construction challenges, but there are a few overarching obstacles to sustained AI growth in the U.S., according to Benjamin Della Rocca, former director for technology & national security at the White House National Security Council.
Regulation. Regulatory barriers are complex for a few main reasons, according to Della Rocca.
- They are site specific
- They involve many government entities
- They have to be addressed at the same time
Permitting happens at federal, state, and local levels, and there are many types of permits, from land use to environmental permits. Depending on where the project is located, some of these can take years to sort out, ultimately slowing AI progress.
There are also additional approvals for transmission projects, like those from state public utility commissions as well as interconnection approvals from utility companies, which can delay project completion for another four to six years, Della Rocca said.
Labor. There is a shortage of skilled workers like electrical workers and engineers who build data centers and transmission infrastructure. The aging U.S. population coupled with too few people entering the trades has led to this drop in skilled labor, according to a 2025 study by McKinsey & Company. The labor shortage is evident with 30% of union electricians approaching retirement age, and the U.S. Bureau of Labor Statistics projects over 80,000 openings for electricians yearly, on average, over the next decade.
Supply chain. Sustaining AI innovation and optimization isn’t limited to graphics processing unit (GPU) access. It’s also reliant on access to components that support AI infrastructure. There’s a domestic shortage of key components for grid technology, including transformers, circuit breakers, cooling racks, and fans. Many of these components are sourced through international supply chains, including from China, adding another layer of complexity amid fluctuating tariffs under the current administration. All of these factors make the physical aspect of AI growth challenging.
What can play a critical role in driving AI innovation in the U.S. forward?
The regulatory environment and energy infrastructure are top of mind as experts consider the factors that can keep the U.S. competitive in AI. To that end, they see the need to make a few foundational investments.
Access to energy, data, and chips. “Whoever has the greatest access to these things at scale is going to be in a position to win the competition for AI leadership,” said Benjamin Schwartz, managing head of infrastructure partnerships and policy at OpenAI. “In this respect, infrastructure is destiny.”
The bottlenecks of AI performance may no longer come from the chips themselves as they have in the past, but from the networking technologies, Breckenfeld added. They may also come from energy-related constraints like connecting multiple data centers together to improve functionality.
“Infrastructure is destiny.”
— Benjamin Schwartz, managing head of infrastructure partnerships and policy at OpenAI
Talent. Investing in education and training around skills that support AI growth in the U.S. will be critical to sustain the country’s lead in AI innovation. This includes technical trades like electrical and grid engineering needed to build the physical infrastructure, as well as STEM fields like computer science, electrical engineering, and energy solutions that will drive technological advancements.
Active government partnership. As with the railroad or interstate system, AI leaders are looking to the government to help back this next big infrastructure project in the U.S. The government can offer support through both investment and regulatory measures to help the country accelerate its AI innovation and stay ahead of major competitors like China that are rapidly expanding their capabilities.