K.T. Ramesh: We need to accelerate and broaden AI research
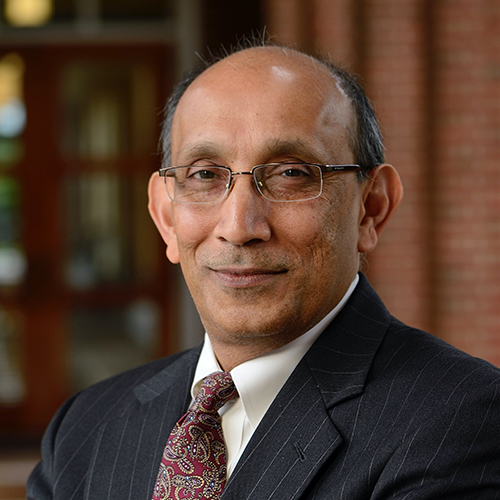
AI researcher and engineer explains how he’s using AI tools and why we need AI regulation
K.T. Ramesh, a professor in Johns Hopkins University’s Department of Mechanical Engineering, has studied everything from materials design to asteroids over his three decades at the university.
He’s now applying artificial intelligence to those areas of study, as well as the mitigation of traumatic brain injury. Additionally, Ramesh is the interim co-director of the JHU Data Science and AI Institute, which is focused on interdisciplinary artificial intelligence and its applications.
We spoke with Ramesh about the institute, his research, and the state of AI more broadly.
You’re using AI to examine such a wide variety of topics, including designing materials for the defense industry, asteroid disruption, and brain injuries. How does AI cut across all these different areas of research?
They’re not actually that different. They’re different applications of the same way of similar thinking. In all cases, my interest is in things that happen quickly—or things that go boom. Whether you’re thinking about a bullet hitting a target or an asteroid hitting the planet, it’s the same basic problem. The difference is the scale, so the way you solve the problem changes because of what kinds of systems you’re talking about, the planet or the brain. But the kinds of equations and the kinds of mathematics are the same.
Then, the AI approach becomes something you can use across these ideas. The way to think about it is you’re solving problems in which many different mechanisms happen together in a short time. AI is great for solving such problems.
What kind of work is the JHU Data Science and AI Institute doing?
The driving vision behind the institute is that with data science and AI, we can address the hard problems that face humanity. You’re able to do big things because you can apply powerful tools that work best on complex problems. For me, the exciting thing is that we can bring many different kinds of experts together, across disciplines, and then use that combination of the experts in each space, together with trusted datasets, to solve some big problems.
For example, one of my colleagues, Natalia Trayanova, is working on an AI system that can predict when someone is likely to have a certain kind of heart problem. Based on what you know about them, looking at their health records up to this point, the syste can assess what the probability is that they will have a cardiac issue of a certain type within the next five to 10 years. That kind of information helps you determine what interventions to make right now. It’s not just for one doctor or patient; any cardiologist will be able to use this kind of system.
We’re also working towards being able to bring AI into the operating room, which could dramatically lower the possibility of errors and increase the quality of service at lower cost. AI can figure out that when this surgeon comes into the OR she will typically want this set of tools, while a different surgeon may want different ones. From there, we can ensure that everything is there when they want them.
We’ve talked a lot about the promise of AI. There are obviously challenges as well. What kind of regulations, if any, do you think policymakers should explore?
Let me start by saying, we absolutely should have regulation in this space. As the tools become more and more powerful, it’s more and more important to know what the impacts will be, to know who has access to those tools, and under what conditions they’re using them.
Some of this can be addressed with voluntary guidelines. But when there are such powerful tools, you need to be sure that there are ways to assess who has done what and to address bad actors. That’s where regulation comes in.
Still, we have to be careful about regulation because if the regulations are too tight, they will stifle innovation, and our economy is based on the fact that we innovate. And we should recognize that it’s not just the U.S. It’s a global competition. Lots of different companies and organizations are growing in the space. If regulations stifle innovation here, things are going to happen in other places anyway.
We have to think about this from the big picture viewpoint that we want to ensure that we have the ability to compete aggressively in the space. And yet we want to place limits on what can be done in some cases.
There’s a big conversation in AI communities right now about if research into artificial intelligence should be accelerated or slowed down. What do you make of that conversation?
It’s a bit of a red herring. I don’t think there’s any way to slow it down this time. It’s in many different forms that grow organically with the availability of data. Given that, there are parts of this research that are going to grow not just here, but in other places. You have to think about accelerating it because you are in a competition, but the key is that you also want to broaden such research. By broaden it I mean, we also want to accelerate the parts of the research system that are thinking about ethics, safety, and alignment with human intent. Those are pieces we have to invest in at the same time.
The danger here is that we slow down the wrong things. What we need to do is ensure that as we’re growing, we’re not thinking in a one-dimensional way.